In many of our previous blogs, we have talked about how artificial intelligence (AI) has been changing the healthcare ecosystem and improving patient outcomes. From enabling more accurate diagnostics, to personalized treatments, AI is allowing researchers to analyze vast quantities of data and make new inferences. However, it is exactly this need for vast amounts of diverse and high-quality data that has proven difficult to achieve. Traditional data centralization methods pose significant challenges, including privacy concerns, data security risks, and regulatory compliance issues, not to mention the associated cost. Additionally to this, health data is often siloed, and it can be hard if not impossible to share it across several institutions. These challenges have lead to the development of a special type of AI models, called federated learning, which we talk about in the next section.
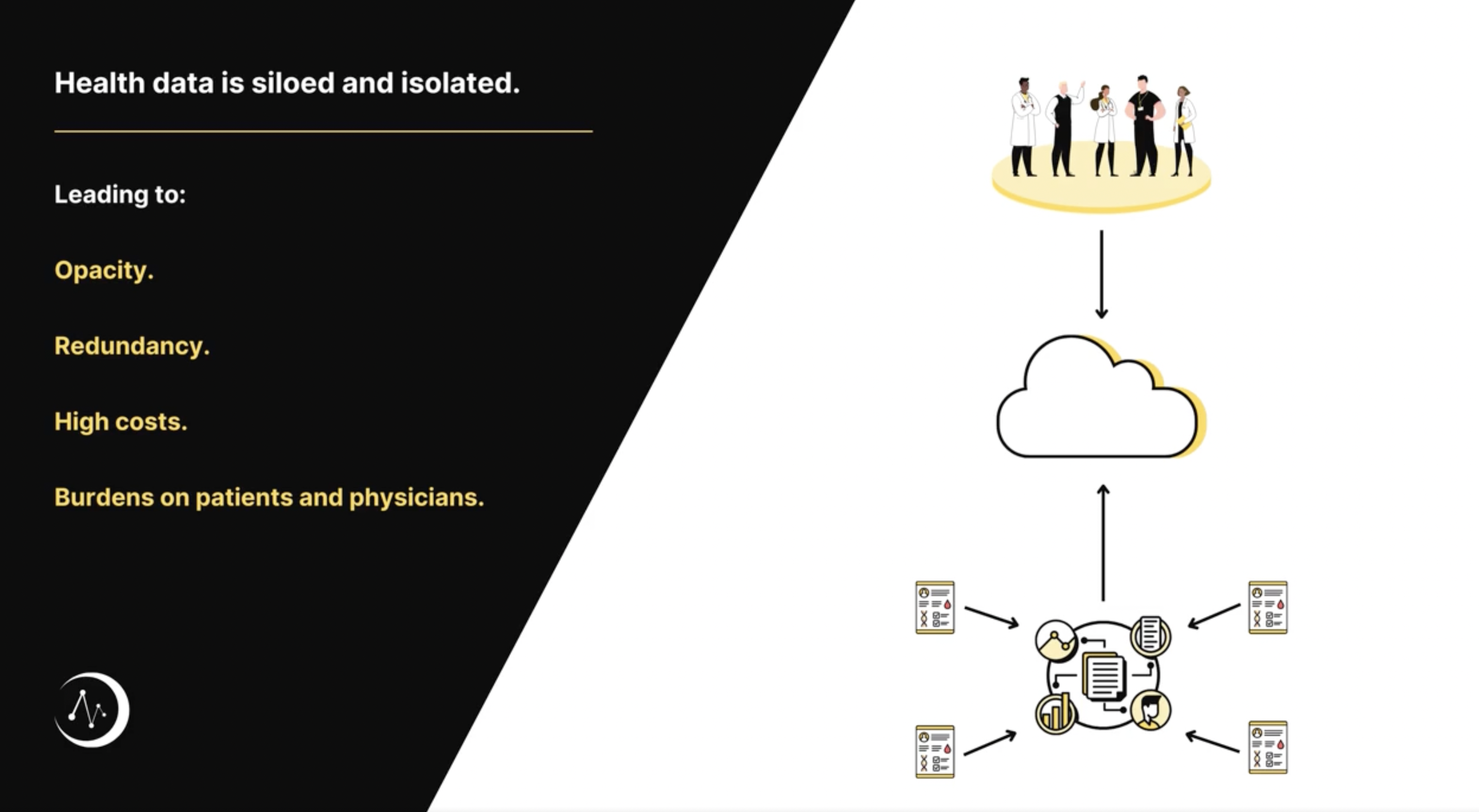
Federated Learning in Healthcare
Federated learning addresses the previously mentioned challenges by allowing AI models to be trained on decentralized data sources, ensuring data privacy and security. This approach enables various healthcare providers to collaborate on AI model training while maintaining stringent data privacy and regulatory compliance, such as HIPAA and GDPR.By training models locally on their individual datasets, federated learning circumvents the need to aggregate sensitive health data into a central repository. Instead, only the learned parameters from each local model are shared and aggregated to create a global model. This method significantly minimizes the risk of data breaches, as patient data never leaves its original location, thus ensuring patient confidentiality is preserved.
The Need for Decentralized and Interoperable Data Access: Cryptographic Data Mesh
As federated learning becomes more popular, the need for decentralized and interoperable access to health data grows. Traditional centralized data storage systems face security vulnerabilities and interoperability challenges, leading to data silos that hinder effective collaboration. Even when data is successfully centralised, the cost of such an approach can be too high. To overcome these issues, healthcare systems must adopt secure, efficient data management solutions that facilitate seamless data integration and sharing.This is why we have created our Cryptographic Data Mesh, an innovative solution that leveraged distributed ledger technology to ensure secure, decentralized data integration. This approach guarantees data integrity, authenticity, and privacy while supporting interoperability between diverse health data systems. The Cryptographic Data Mesh allows healthcare providers to access and share health data securely and efficiently, eliminating the need for costly and complex data migrations and enhancing trust and collaboration among healthcare entities. Some of the benefits include:
1. Enhanced Privacy and Security: Ensures that sensitive health data remains protected.
2. Improved Data Interoperability: Facilitates better collaboration and data sharing across different systems.
3. Regulatory Compliance: Adheres to stringent data privacy regulations, reducing legal risks.
4. Cost Efficiency: Reduces costs associated with data migration and infrastructure changes.
5. Increased Trust: Cryptographic validation of data enhances trust among healthcare providers and patients.
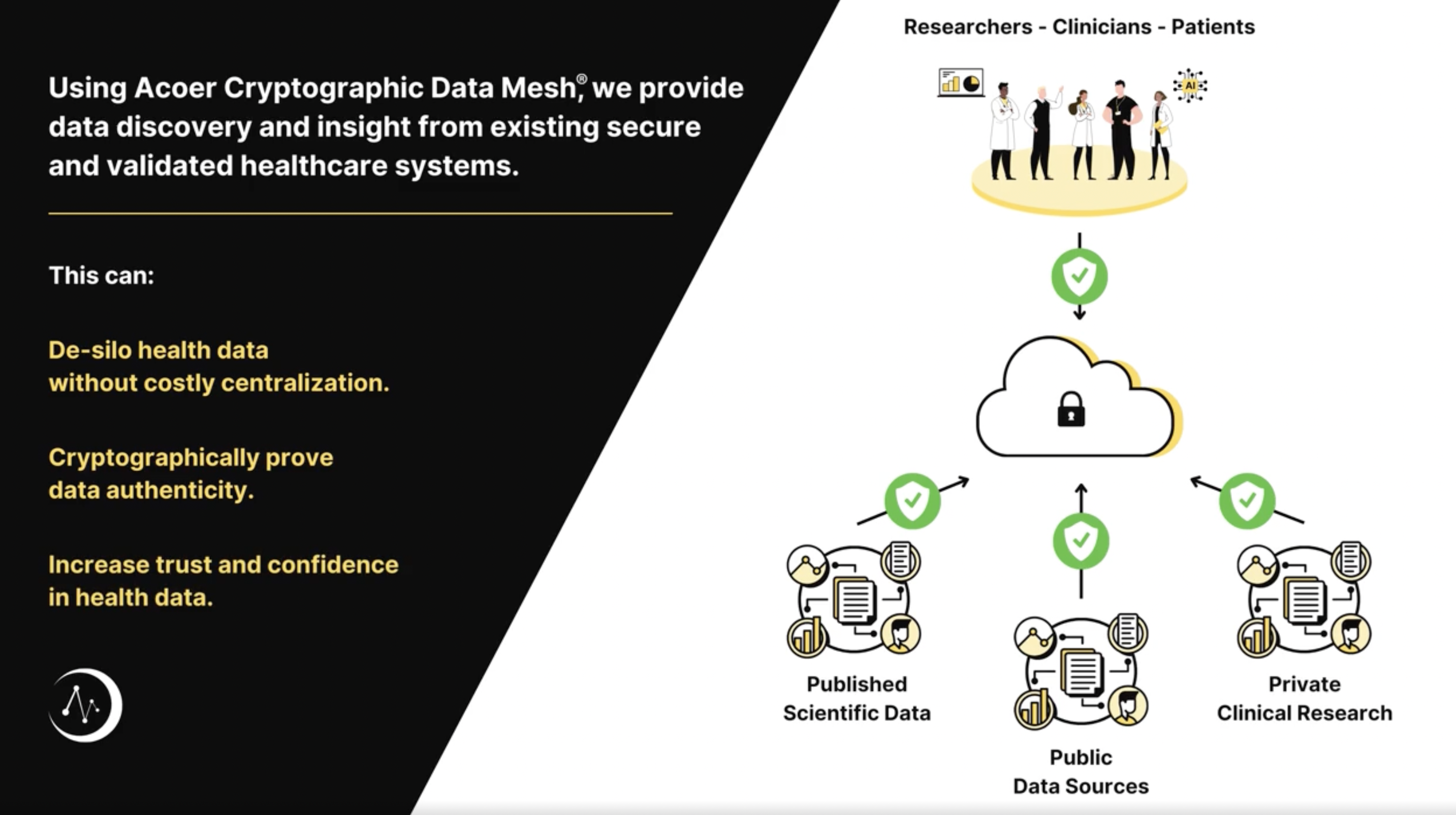
Federated learning, combined with decentralized and interoperable data access solutions like our Cryptographic Data Mesh, represents the future of AI in healthcare. By enhancing data security, promoting fairness, and ensuring transparency, the Cryptographic Data Mesh contributes significantly to the ethical application of AI in healthcare, ultimately improving patient outcomes and fostering trust in AI-driven healthcare solutions. You can learn more about Cryptographic Data Mesh here or reach out to our team if you have any questions.